Mistakes to Avoid: Common Pitfalls in the Scientific Analysis of Biological and Chemical Data
The field of biology and chemistry presents a complex landscape of data analysis. Whether you're a new scientific analyst or a seasoned expert, navigating the intricate world of biological and chemical data demands precision and attention to detail. However, it's not uncommon to stumble upon pitfalls that can skew results or lead to incorrect conclusions. This guide highlights common mistakes made during the scientific analysis of biological and chemical data and offers strategies to avoid them.
Understanding the Significance of Accurate Data Analysis
Before diving into the mistakes, let's emphasize the importance of accurate data analysis. In both biological and chemical sciences, accurate data analysis is crucial for credible research findings. Misinterpreted data can lead to erroneous scientific conclusions, potentially hampering scientific progress and clinical applications. It serves as the backbone for effective experimentation, hypothesis testing, and peer-reviewed publications.
Common Pitfalls in Biological Data Analysis
1. Neglecting Proper Data Collection Methods
One of the frequent errors in biological data analysis is overlooking robust data collection methodologies. Ensuring that data collected aligns with the research question and hypothesis is fundamental. Often, analysts may rush through this phase, leading to incomplete or biased data, which directly affects the analysis phase.
Solution: Adhere to standardized protocols for data collection. Quality control measures, such as calibrating instruments and validating procedures, should be routinely implemented.
2. Inadequate Sample Size and Power
Sample size and statistical power are essential in driving reliable conclusions. Small sample sizes can lead to inconclusive results and reduce the statistical power of your study.
Solution: Initially determine the appropriate sample size using power analysis. This step assures that results are statistically significant and reflective of the population.
3. Disregarding Data Cleaning and Preprocessing
Biological data often contain outliers, missing values, and noise which can obscure true patterns and associations if not properly addressed.
Solution: Implement rigorous data cleaning procedures, including handling missing data through imputation or exclusion, and normalizing data distributions.
4. Misinterpretation of Statistical Results
Statistical tests can yield complex results that, if misinterpreted, can result in wrong conclusions. Analysts often make mistakes such as confusing correlation with causation.
Solution: Utilize clear statistical interpretations and consider consulting statisticians or software tools that offer step-by-step analysis and output explanation.
Common Pitfalls in Chemical Data Analysis
1. Failure to Calibrate Analytical Instruments
Analytical equipment must be regularly calibrated for accurate chemical data analysis. Failure to do so can lead to systematic errors.
Solution: Implement regular calibration schedules to maintain the precision and accuracy of measuring instruments.
2. Ignoring Reactant and Product Interference
Chemical reactions often involve substances that can interfere with analysis, potentially leading to erroneous chemical interpretation.
Solution: Apply appropriate controls and matrices to account for interference and ensure that all variables are considered in the analysis.
3. Inadequate Sophisticated Software Utilization
Many scientific analysts fail to take full advantage of sophisticated chemical analysis software, resulting in inefficient or inaccurate analysis processes.
Solution: Gain proficiency in specialized software tools that can process complex data accurately and swiftly, facilitating a more nuanced understanding of results.
Universal Mistakes Across Biological and Chemical Data Analysis
1. Lack of Reproducibility
Reproducibility is a cornerstone of scientific research. An inability to replicate results in independent labs signifies potential issues in the analysis process or data integrity.
Solution: Document methodologies meticulously to ensure that other researchers can replicate your study with the same outcomes.
2. Incomplete Documentation
Accurate records of every step in the analysis process are essential. Many scientists omit thorough documentation, which is crucial for reproducibility and peer verification.
Solution: Maintain comprehensive records of all experimental procedures, data collection methods, and analysis techniques.
3. Overlooking Ethical Considerations
Data analysis often raises valuable ethical considerations, particularly concerning data privacy and consent in biological studies.
Solution: Ensure compliance with ethical standards and institution review boards (IRBs) to safeguard participant data and research integrity.
Conclusion: Improving Scientific Data Analysis
Enhancing the quality of biological and chemical data analysis requires vigilance, precision, and ongoing learning. By recognizing and circumventing common pitfalls, scientific analysts can foster more reliable, meaningful scientific discovery. Proper data management, statistical literacy, and ethical mindfulness are the backbones of sound analysis. As the landscape of scientific research evolves, so too must the practices guiding rational data interpretation.
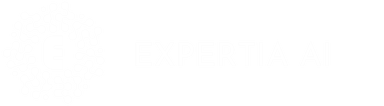
Made with from India for the World
Bangalore 560101
© 2025 Expertia AI. Copyright and rights reserved
© 2025 Expertia AI. Copyright and rights reserved